A07 – Machine Learning for Defect Phases
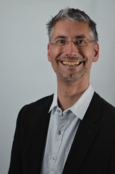

PI(s): Ulrich Kerzel (RWTH)
SFB researchers: Khalil Rejiba (RWTH)
Electron backscattering diffraction (EBSD) analysis is an invaluable tool for the analysis of the structure in polycrystalline materials. The first results using machine learning reported in the literature have shown the potential of using these methods in EBSD analysis. Furthermore, recent advances in sensor hardware, such as the TimePix sensor, allow to measure the energy of the incident electrons, allowing for further improvements of the acquired data and their subsequent analysis. The aim of this project is to develop novel methods for EBSD analysis based on recent advances in machine learning and computer vision, as well as to exploit more detailed information about incident electrons.
A key aspect is the use of explainable machine learning techniques to connect the models of these approaches with the experience of human experts grounded in a detailed understanding of the underlying physical processes. The use of machine learning requires large amounts of simulated and experimental data. Additionally, a wide range of diverse metadata need to be captured from simulation, data acquisition, and machine learning training and inference. Similarly, the various research groups in this CRC each have their own unique and diverse requirements for capturing, storing, processing, and sharing data. Therefore, the second focus of this project is to develop a comprehensive research data management system that allows the storage and processing of all data and meta-data from the research groups of this CRC. Building on open standards and established software systems, this will allow researchers not only to capture, store, and process their data and share them within the CRC, but also enable them to publish relevant data in public repositories for the wider scientific research community. Including best practices from software development standards will, in addition, allow to establish data-centric workflows that can, in part, be automatef.
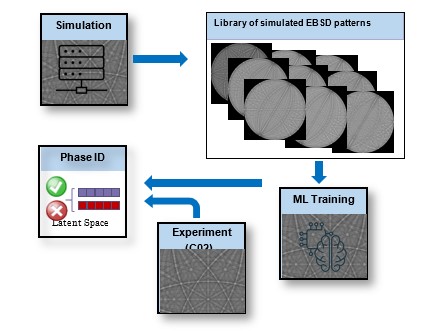